

Memory Specifications Specification Tesla PCIe 16GB Tesla PCIe 12GB Maximum memory clock 715 MHz 715 MHz Memory size 16GB HBM2 12GB HBM2 Memory bus width 4096-bit 3072-bit Peak memory bandwidth Up to 732 GB/s Up to 549 GB/s Table 3 provides the software specifications that apply to both the 16GB and the 12GB. Product Specifications Specification 16GB 12GB Product SKUs NVPN: 699-2H XXX NVPN: 699-2H XXX Total board power 250 W GPU SKUs GP A1 GP A1 PCI Device IDs Device ID: 0x15F8 Vendor ID: 0x10DE Sub-Vendor ID: 0x10DE Sub-System ID: 0x118F Device ID: 0x15F7 Vendor ID: 0x10DE Sub-Vendor ID: 0x10DE Sub-System ID: 0x11DA NVIDIA CUDA cores 3584 GPU Base 1189 MHz clocks Maximum boost 1328 MHz VBIOS EEPROM size 4 Mbit UEFI Supported PCI Express interfaces PCI Express Lane and polarity reversal supported Power connectors and headers One CPU 8-pin auxiliary power connector Board 1177 Grams Weight Bracket with screws 21 Grams Long offset extender 52 Grams Straight extender 42 Grams GPU Accelerator PB _v01 2ħ Specifications Table 2 provides the memory specifications for the board. GPU Accelerator PB _v01 1Ħ SPECIFICATIONS PRODUCT SPECIFICATIONS Table 1 provides the product specifications for the GPU Accelerator. The Tesla P100 for PCIe is available in two memory configurations: with 16GB HBM2 with 12GB HBM2 As a board design, both the memory configurations are identical and this product brief covers both configurations. For more information on compute capabilities, HBM2, unified virtual memory, and page migration engine visit NVIDIA official website. The with HBM2 memory has native support for ECC and has no ECC overhead, both in memory capacity and bandwidth.

ECC protects the DRAM content by fixing any single-bit errors and detecting double-bit errors. The GPU will replay any memory transaction that have an ECC error until the data transfer is error-free. ECC protects the memory interface by detecting any single, double, and all odd-bit errors.

boards are shipped with ECC enabled by default to protect the GPU s memory interface and the on-board memories. By adjusting the GPU clock dynamically, maximum performance is achieved within the power cap limit. For performance optimization, NVIDIA GPU Boost feature is supported. The supports double precision (FP64), single precision (FP32) and half precision (FP16) compute tasks, unified virtual memory and page migration engine. It uses a passive heat sink for cooling, which requires system air flow to properly operate the card within its thermal limits. Languages Supported GPU Accelerator PB _v01 ivĥ OVERVIEW The NVIDIA Tesla P100 GPU Accelerator for PCIe is a dual-slot 10.5 inch PCI Express Gen3 card with a single NVIDIA Pascal GP100 graphics processing unit (GPU). Minimum CFM Requirements per Inlet Air Temperature. Board Environment and Reliability Specifications. Straight Extender LIST OF TABLES Table 1. CPU 8-Pin Power Connector with Optional I/O Bracket. Board Dimensions with Optional I/O Bracket. Airflow Directions with Optional I/O Bracket.
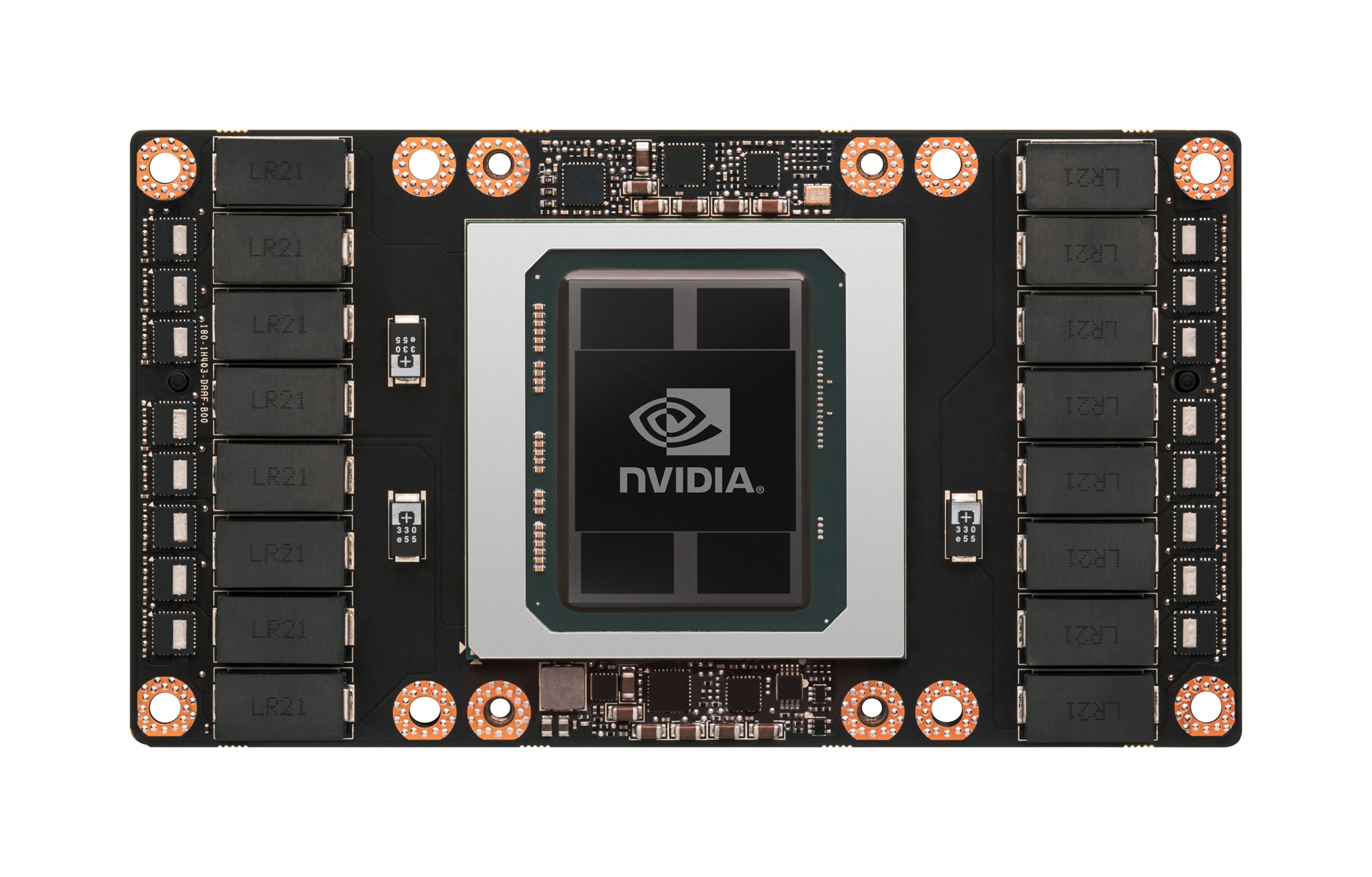
9 Support Information Certificates and Agencies Certifications Agencies Languages GPU Accelerator PB _v01 iiiĤ LIST OF FIGURES Figure 1. Or it's simply a 2x4x2 in one clock and I'm reading too much into a diagram.1 TESLA P100 PCIe GPU ACCELERATOR PB _v01 October 2016 Product BriefĢ DOCUMENT CHANGE HISTORY PB _v01 Version Date Authors Description of Change 01 OctoGG, SM Initial Release GPU Accelerator PB _v01 iiģ TABLE OF CONTENTS Overview. It might actually be doing a 2x4x4 over two clocks, in which case you might be able to alternate vector and tensor instructions? But then I suspect nvidia would be advertising it as 30 TFLOPS FP64. Which I normally wouldn't really nitpick but all the other precisions are depicted doing valid multiplications. In the white paper nvidia depicts fp64 multiplying two 2x4 matrices which isn't a valid matrix multiplication (for AxB the # of columns of A must match the number of rows of B). With fp64 tensors only executing at double the rate, though, it might only be doing one instruction per 2 clocks. Most tensor core operations block everything else as they hog all available instruction and register file bandwidth. Why include CUDA cores FP64 at all if tensors are way faster?īecause you might have a use case for fp64 that isn't matrix multiplication?Ĭan they be used simultaneously, as in added together to give us 29TFLOPs of FP64 performance?
